Social Media for Predicting the Mexican Presidential Election: Beyond X and Facebook
DOI: https://doi.org/10.1145/3680127.3680213
ICEGOV 2024: 17th International Conference on Theory and Practice of Electronic Governance, Pretoria, South Africa, October 2024
The surge of social media usage and its importance on political campaigns across the globe has been imminent. As new electoral processes begin, new social media tactics appear each time. This behavior does not exclude Latin American countries and candidates. In response, recent research has analyzed how these platforms may aid in the search for new and scalable methodologies to model voting preference from social media like traditional pollsters do. However, most of the literature does not consider multiple networks and delimits its scope to the analysis of sentiment classification in either Facebook or X (formerly Twitter). In this way, the current research aims to study how mostly ignored social media apps like Instagram and YouTube can change the outcome of predictions online. We consider the case of the 2024 Mexican Presidential election and how social media behavior relates to what the polls say about the candidates. We conclude that Instagram and YouTube can turn failed predictors into success by considering the importance that features from these platforms provide to machine learning models.
ACM Reference Format:
Hector Abel Gutierrez, Alejandro De León, Mahdi Zareei, and Kellyton dos Santos. 2024. Social Media for Predicting the Mexican Presidential Election: Beyond X and Facebook. In 17th International Conference on Theory and Practice of Electronic Governance (ICEGOV 2024), October 01--04, 2024, Pretoria, South Africa. ACM, New York, NY, USA 10 Pages. https://doi.org/10.1145/3680127.3680213
1 Introduction
Polling, and more specifically in Mexico, has been a subject of concern to federal authorities, as was evidenced in the 2023 elections held in Estado de México, México: The “Tribunal Electoral del Poder Judicial de la Federación”, México´s biggest authority in electoral matters, sanctioned 8 pollsters due to the lack of support for their methodology [25]. They all gave the party in power's candidate, Delfina Gómez, a considerably large margin of victory of over 15%. These results were used by her team to argue it was evident "8 out of 10 wanted the change" [9], a strategy named the “bandwagon effect", in which a conceived behavior of many can affect the behavior of the individuals [15]. As election day arrived, the final difference was only 8 points. More importantly, only 50% of the total electorate voted in the election [13].
The final difference between the official result of the election and the previous predictions by the polls is another example of why people have lost faith in polling processes [7] [22]. Based on cases like this is that social media (SM) has been regarded as a theoretical framework to overtake traditional polling methods due to its outreach, speed of iteration, and cost. More specifically, it is presumed that social media can provide a procedural methodology for scientists to demonstrate the source of their polling results, as not only does it provide millions of data points on opinions and concerns from the general public, but it has also allowed a variety of studies to conclude it can reflect a general political stance [6].
Different social media applications like YouTube, have emerged as the way the Mexican president provides the official news set to his viewers, even making him a highly-ranked streamer ever since [2]. This sets an important precedence to how modern social media applications are trusted by Mexican figures to reach millions of users.
But of all the available social media platforms today, X (formerly Twitter) has produced the most amount of research in the field. Not only because of its increasing number of users but because it has been regarded as the official communication platform for many political figures [24].
It is in this way that Twitter has been subject to many types of analysis trying to prove whether it may be considered a viable option to represent a voting population or not. More specifically, Twitter has been used to track how people feel about a candidate and its adversary: the ultimate purpose of an election. For this, the usage of machine learning via sentiment analysis (predicting the sentiment in an abstract of text or group of texts) has been the most used approach to try to mimic an electoral public [[28] [16]. While this has been validated by many, it has also received criticism due to the lack of involvement from ’real’ indicators like polling results or the inclusion of the sentiment from people posting on other social media apps like Facebook or Instagram [12]. In addition, there has been a disproportionate amount of research regarding the usage of sentiment analysis in Latin American elections compared to predicting those in the USA. However, as proposed in [6] and extended in [4], a shift from the typical sentiment-analysis-based approach can result in satisfactory predictive results. These indicate that transforming the problem of modeling via sentiment analysis of how people feel on Twitter evolves into counting how many people pay direct attention to each candidate and their posts on social media, creating a ’snowball’ effect and propagating the message to even more users systematically [11]. In other words, it is a viable option to credit these challenges as regression problems (predicting a numeric value) to trace the number of interactions a candidate has in social media with their electoral preference rather than those based on overall sentiment classification. However, both X and Facebook are still the platforms with the most attention for this manner while others may still play a crucial role in predicting the overall preference of the people.
Based on the cited work which is taken as the base of this research [4], the aim of the current study is, therefore: i) to model the likeliness that social media apps used by the 2024 Mexican presidential election candidates reflect the current state of the polls and ii) to evidence the importance that features within YouTube and Instagram (highly-ignored by the literature apps) may play in the prediction of a voting preference with the usage of machine learning models. To do so, we aim to evidence the weighing and importance these features have when predicting and modeling the current state of the polls and contrast these with those that come from X and Facebook. In other words, are features coming from Instagram and YouTube affecting or influencing voting predictions, and if so, how do these differ from those used by most of the literature: features from X and Facebook?
The remainder of the work is organized as follows. Section 2 provides an overview of the current state of the Mexican presidential election, to be held on the second day of June 2024. Section 3 illustrates the data collection process and experiments and section 4 provides their results to answer the proposed questions. Finally, this paper concludes with an analysis of the results and points out the major takeaways from this research.
2 Background
2.1 The Current Mexican Election
Describing Mexico´s electoral process, presidential elections are held every six years. In 2024, they will take place on June 2. The party in power, MORENA, has already defined its candidate with anticipation: former Mexico City´s state chief Claudia Sheinbaum. In the same sense, the opposition side has made official their trust in federal senator Xóchitl Gálvez to represent their 3-party alliance: PRI, PAN, and PRD. In a sudden turn of events, the other major party that remained unrepresented, Movimiento Ciudadano, originally decided to give Nuevo Leon´s governor Samuel García their support. Still, after some state-related conflicts around García´s current role, Movimiento Ciudadano opted for federal senator Jorge Álvarez Maynez.
The race between Gálvez and Sheinbaum, the two main candidates in the election, was officially announced on September 7 (when Sheinbaum was declared the candidate for MORENA), the major and most trusted news sites in Mexico in accordance to the 2022 Digital News Report by Reuters had Sheinbaum in a far-off first place with around 50% of the preference, Gálvez reaching 25% of the preference, and García with around 10%, except for unique cases of polls not ranked by Reuters which had Movimiento Ciudadano's candidate with more than 20% of the voting preference and Gálvez with close to 15% [23], noting the case for this study.
Mexico´s official electoral authority, INE (Instituto Nacional Electoral), has approved 19 polling institutions [10] to conduct polls for the 2024 electoral process. However, results from other organizations are used regardless of their credited status by the current candidates in campaigns. It is key in this case to identify the source of each result shown.
In the current study, we will refer to the analyzed polls as those published by Oraculus. mx [[19] which is a credited and independent organization in charge of ’polling the polls’ or contrasting their results to form a single indicator from all of them. Their public and documented selection process to include a poll or not is based on credibility by experts in the subject. Their output list will be selected to perform all the experiments for the next sections in this work. In the same sense, its constructed prediction will be used to contrast the set of predicted results by the current study. Their founders, Jorge Buendía, Juan Ricardo Pérez Escamilla, Javier Márquez, and Leonardo Zuckerman are renamed and well-acknowledged journalists in Mexico and created Oraculus to propose a new methodology to traditional polling in the country.
2.2 Related Work and Research Questions
2.2.1 Preceding Work. For the correct assessment of the current research, it is important to state that we aim to continue the progress performed by Brito et. al. [4], in which several Latin American elections were modeled, tracked, and forecasted with different machine learning algorithms. Unlike the other included countries, Mexico was modeled by only including Facebook and X, due to the lack of data from official Instagram profiles of Mexican candidates.
In the commented work, Brito et. al. constructed different regression algorithms that were proven to correctly predict election results: a base linear regression (LR) model, a multi-layer perceptron model (MLP), and recurrent neural networks. This provided a different methodology to challenge the established sentiment analysis background in the field: regression to monitor social media interactions within the candidates’ profiles, as mentioned previously.
This approach bases its theory on [18] and [29] which mention that "the mere repeated exposure of the individual to a stimulus object enhances his attitude towards it”, also referenced by [30] which correlates the political exposure of candidates with their electoral performance. With this, we can analyze the attention gathered by the candidates and reference a regression problem focusing the analysis on selected metrics that dictate the attention they get through their social media presence.
Similarly, the extracted data in the mentioned work was pre-processed with the PCA algorithm (Principal Component Analysis), a technique used to reduce the number of features (columns) in a dataset to model a prediction with each algorithm via existing libraries, as will be explained in upcoming sections. Another important consideration is that the PCA algorithm automatically scales the data before projecting it in fewer dimensions, with this, the current work will only scale the newly gathered data and will not apply PCA since the data we´ll work with is ’small’ enough to allow the integration of all the considered columns, in contrast to [4].
As previously discussed, most of the current research in the field revolves around targeting X (Twitter) as the only source of information or the base of targeted databases for modeling. In addition to this, not only is X considered as a sole source for data, but also a single approach to model a polling percentage is targeted: volume sentiment analysis, as discussed in [20], [17], and [12].
Nevertheless, it is important to state a clear picture of the state of the research in which social media is used to predict elections not only in Mexico but globally. As researched by [6], social media usage in electoral contexts can be subdivided into three main categories:
- The electoral context in which the different studies are being performed
- The different types of approaches that are used to try to target the voting percentage within an election with social media
- The characteristics that can define a successful study in the field
We´ll explore the existing literature for predicting elections following this categorical division and include a subsection for related work targeting to find a correlation between social media performance from a set of candidates and their actual electoral results.
2.2.2 Electoral context behind social-media-based electoral predictions. As studied in [17] and [12], social media usage is considered around a single election process. This results in the majority of cases for studies of this type: a single election process which is later difficultly replicated in multi-election studies. In addition, most of the studies analyzed (68%), focused on the context of national elections [6], followed by state and local election processes thereafter. Also, it is reviewed that a great share of studies analyzed the voting percentage a candidate received, other than a party. As a major shift in the context of the current work, 42% of the studies considered a voting process of only two candidates, this is greatly influenced by the majority of research around the US presidential campaign and federal processes in the country. Only 30% of studies considered a 3-5 candidate election, which is the case for this research, with Sheinbaum, Gálvez, and Máinez.
2.2.3 Different approaches to predicting elections with social media. As concluded in 2021 and revised in early 2024, only 7 studies compared more than two social media platforms with Twitter and Facebook to integrate machine learning models (6%) and predict elections with social media, when contrasted with those relying solely on Twitter (62 studies); as shown in Table 1, from [6].
Social Networks used as Data Input | Studies | (%) |
---|---|---|
Only Twitter | 62 | 75 |
Only Facebook | 7 | 8 |
Twitter, Facebook, and others | 6 | 7 |
Twitter and others | 2 | 2 |
Facebook and others | 2 | 2 |
Others | 4 | 5 |
It is clear in this case a great gap in the use of a single social media network to try to predict the outcome of an election. There is no clear evidence of the reliance on Twitter as a sole source of information for this case. This is more evident when considering different studies evidencing Twitter as not only not the most-used social media application, but smaller in comparison to other feasible options like Facebook or Instagram [5] (both to be analyzed in the current work).
In regards to the existing data collection processes proposed by the literature and analyzed by [6], the strategy remains constant: a search through the platform´s posts via application programming interfaces (APIs) with the usage of predefined keywords and hashtags that can match electoral contexts. These metrics are predefined by the research team arbitrarily and exposed in the literature. This has caused controversy in the field, as keywords and hashtags are the main strategy when trying to capture the most amount of data points for the research, if predefined incorrectly, the strategy perishes.
When in discussion about the modeling strategy, 77% of the analyzed research performed a volume-based sentiment analysis approach, this is, targeting the most amount of tweets that talked about or discussed a certain keyword or hashtag that matched with the research´s parameters. The process can be simplified as follows:
- data selection based on keywords and hashtags
- excluding repeated information and which did not target the predefined parameters
- sentiment analysis performance of the resulting database
- performance evaluation
2.2.4 Characteristics of success. Brito et. al. [6] defined ’successful’ research to be the one that could report results consisting of a Mean Absolute Error (MAE) of 2% or lower between the prediction of the election and its actual result, this was based on [14], which performed a comparative study of more than 30,000 polls between 1942 and 2017, and concluded a historical MAE of 2.7%. Unsuccessful research was considered to have an MAE above 6%.
Only 63% of studies could pass the 2.7% mark, and only 55% of studies conducting volume sentiment analysis could obtain a better score than a 2% margin of error. 72% of the studies that used a regression analysis found success in their results, an important detail since this is the implemented approach throughout the present work. Another important discovery lies in the usage of solely Facebook, resulting in 80% of their studies finding success [6].
Twitter was not a definite synonym for success in the case of predicting elections, nor was it the usage of the common sentiment analysis processes as exposed previously.
2.2.5 Correlation analysis for social media presence and electoral results. Apart from modeling the results of an electoral process with social media data, there have been various studies aiming to find a direct relationship between social media metrics and election results. In 2010, Tamusjan et. al. [26] concluded that the relative volumes of tweets closely mirrored the federal German election results. Three years later, DiGrazia et. al. [8] found a significant correlation between the number of tweets mentioning a candidate for the U.S. House of Representatives and the electoral result of the mentioned candidate. Moreover, in a study made to target the 2018 Mexican elections among other Latin American countries too, Brito et. al. [3] concluded that the metrics presented in the current study, to be later presented, showed a correlation with the result of the election regardless of the starting collection date.
The importance of this correlation analysis for the current study is to aim to find a relationship between what social media activity reflects for the current candidates and what the considered set of polls predicts will happen. Do they tell the same story? This is particularly of interest since the results of the 2024 election are still unknown and if a correlation is found, we´ll be able to state if they were both completely wrong after results are known. If they differ completely in the direction of each candidate, we´ll be able to analyze which of these (SM or polls) were correct and better reflected the actual state of the election.
Therefore, based on the material and the current state of the research, the following are then considered to be the questions to be targeted with the following study:
- RQ1. Which social media features are correlated the most with the electoral performance of the selected candidates?
- RQ2. Do features from Instagram and YouTube, which are overlooked by most of the current research, change the outcome of a set of predictions towards the presidential election in Mexico?
3 Data and methodology
By now, we´ve illustrated how the current Mexican election has evolved from early September. Also, we defined the current state of the literature on the topic. Throughout the following section, we´ll explain how we handled the process of translating the problem from the commonly-used approach of sentiment analysis to try to predict an election result, to utilizing regression algorithms and considering both offline and online indicators to build predictors and answer the proposed research questions.
As proposed by [4], we constructed a dataset that considered the daily state of interactions that Sheinbaum, Gálvez, and Maynez had on their social media profiles. To make this more concise, we collected the total number of posts, likes, shares, and comments the three candidates had on Twitter, Facebook, Instagram, and YouTube.
It´s important to remark that the name of these different metrics may vary from one platform to another: i) likes, comments, and RTs for X (Twitter), ii) reactions, comments, and shares for Facebook, iii) likes for Instagram (comments were excluded as a period in the platform denied access to these), and iv) views for YouTube. To be more precise, a list of all the feature names in each social media app is listed below:
- XPosts: Number of posts on X
- XComments: Number of comments on X
- XRts: Number of rts on X
- XLikes: Number of likes on X
- XCommsPPost: Number of comments per post on X
- XRtsPPost: Number of rts per post on X
- XLikesPPost: Number of likes per post on X
- FBPosts: Number of posts on Facebook
- FBReactions: Number of reactions on Facebook
- FBFBComments: Number of comments on Facebook
- FBShares: Number of shares in Facebook
- FBReactsPPost: Number of reactions per post on FB
- FBCommsPPost: Number of comments per post on FB
- FBSharesPPost: Number of shares per post on FB
- IGPosts: Number of posts on Instagram
- IGLikes: Number of likes on Instagram
- IGLikesPPost: Number of likes per post on IG
- YTPosts: Number of posts on YouTube
- YTViews: Number of views on YouTube
- YTViewsPPost: Number of views per post on YT
3.0.6 Dataset. The dataset construction was performed daily, as we manually analyzed and retrieved the mentioned metrics directly from the candidates’ profiles in all social media platforms discussed. This process ensured the correct representation of the actual posts from the candidates and a precise metric count to be included in the dataset, which has also become a risk in the modern literature when considering recent rate limits implemented by X [5]. Because the construction of the dataset was planned before the candidates were announced, this labor could be handled manually. However, the data collection process can also be performed via the official API (Application Programming Interface) of each social media application. This allows the retrieval of data at any point in time. As also exposed in [5], recent-surging API prices also propose an impediment to data retrieval processes from social media apps, especially X and new alternatives should be considered if this approach is selected.
Since the process was followed each day, we allowed one week for the data to ’settle’, meaning the listed metrics were retrieved one week after the post they belonged to was posted. This period was determined with metric observations to analyze a clear stop in user interaction with a post regarding each candidate. Figure 1 outputs the number of comments in a sample post for both Sheinbaum and Gálvez for a series of days, in it we can see a clear plateau after the fifth day.
In the same sense, a daily average of each parameter was performed regarding a single post: in addition to the number of posts, likes, and comments for a certain candidate on a given day, we would average the number of likes, comments, and shares that a single post would have on that day. A sample of this can be found in Figure 2.
As the database was periodically constructed, we considered 10 different periods from which we would decide to sum these results depending on polling announcement dates: 1 through 7, 14, 21, and 28. In other words, each time a selected poll published results, we would construct 10 different datasets (from the periods mentioned) for each candidate summing the total number of each feature (excluding PPost features) and averaging the number of these that were present through that time frame to fill the PPOST features. The process is depicted as follows:
- A daily collection for the number of different parameters and their daily averages for that day (Figure 2)
- Collect the polling performance for each candidate (% of preference) and the date of each major poll released
- Sum each category of parameters based on the ten different periods previously defined (1..7, 14, 21, 28) and average these through the analyzed period.
- 10 different datasets for each candidate consisting of 10 different summed-up days would feed regression models.
3.1 Polls Included
Around the polls considered, this proposed a major challenge. In the Mexican election context, there are no predefined guidelines to assign a trust level to each organization that may perform a polling process and publish results. For this, we considered only those that Oraculus.mx [19] published on its official site, as previously discussed. Since Oraculus does not provide a concise day for each published poll but rather only the month in which they were performed, we assigned the collected polls to be conducted starting on the 15th of each month. This is not a particular detail of interest, but rather a reference guideline to start counting the predefined set of days. e.g. the first poll was labeled on the 15th, the second on the 16th, and so on.
As previously discussed, the general trust in the polling system has encountered a decline. In the same sense, recent sanctions and predictive errors have also contributed to damage the collective trust in the polls. For this, the current work takes Oraculus as a solution: while we take polling results as a part of the modeling and predictive processes to prove machine learning models along with real-world indicators (polls in this case) can outperform traditional methodologies, we only consider highly evaluated and studied pollsters that were previously analyzed and filtered by the Oraculus.mx team based on historic results. Since we praise the inclusion of offline features to contribute to the model, and not only consider those from social media or online platforms, the inclusion of polls in this case is needed. We support this in the evaluation section also.
3.2 Modeling
As the final consideration for the current work, the linear regression and the multi-layer perceptron algorithms are used to model polling results, like in [4], and following the depicted set of hyper-parameters described in the research to be latterly described. Although various regression models can be used to try to predict the result of the 2024 Mexican presidential election in further research, the principal focus of the present work is to prove polling behavior via regression analysis can be both replicated and predicted, while also analyzing which features of the described dataset may be more important for this process and why it may be the case.
In addition to the modeling selection, the incoming data will be modeled with the usage of scaling. We will be referring to standard scaling as detailed in the Scikit-learn documentation [21]. Principal Component Analysis (PCA) was discussed in [4] as an alternative to avoid dimensionality problems as exposed in [27]. However, since we can not ensure a linear relationship between all the features included and the objective of the experiment is the analysis of the importance each social media platform may have in expected predictions, PCA will be discarded as a dimensionality-reduction technique, as it has been stated multiple platforms should be considered for predictions.
Finally, we´ll discuss the integration of all the predictions made for each time frame (1...7,14,21, and 28 days). An ensemble of predictors was created for this set of experiments. Each model (LR and MLP) is trained on a different dataset consisting of each selected period before the poll is considered. This results in 10 different predictions for each candidate. These results are then averaged to form a single, predicted result. This was based on the methodology in [4].
Moreover, the predictions and the overall process can be described as follows:
- Build a database that outputs the number of features before each time frame for each poll. The number of rows in each dataset represents a poll from September 2023 to January 2024 (see Figure 3 for an extract)
- 10 different datasets were considered for each candidate (since we count with 10 different time-frames)
- Both ten LR models and ten MLP models are trained with each of the databases mentioned above.
- We construct two instances of each model since we consider both all features and only X, and Facebook features
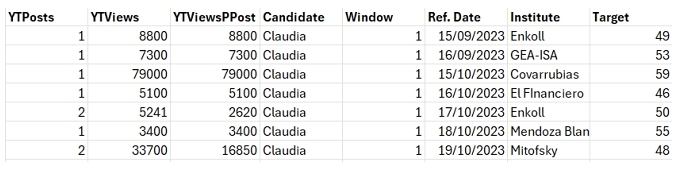
3.3 Evaluation
To evaluate RQ1, we calculated the Pearson correlation coefficient r between the social media metrics selected and the corresponding voting preference depicted by the polls. In this case, since we still don´t count on a unique reference to guide our evaluations (as a final election result would be), we considered the average percentage of preference for each month for each candidate. This is, we obtained the monthly mean of all the included polling results, compared it with the social media performance of every candidate, and analyzed the correlation between those metrics up to the last day of January. The coefficient r may vary from -1 to 1. A value of 1 would mean that as each variable increases the other one does too, depicting a linear correlation. A value of -1 means there exists a perfect negative correlation between the two variables and a zero value translates into not being correlated at all. More specifically, we considered a value for r to be greater than 0.7 to conclude a high correlation exists between social media performance and polling results, following the commonly accepted margin.
For RQ2, two predictions for each model are performed: i) one considering a dataset constructed by features only from X and Facebook and ii) a prediction based on datasets formed by features from all social media applications. Moreover, Mean Absolute Error (MAE) is reported for each model in contrast with Oraculus´s results.
To evaluate the importance of the included features and their role in a predicted result, we analyzed the feature importance for each model after the first round of experiments that included only features belonging to X and Facebook. After this, we performed the feature importance evaluation for the models including all features from all social media platforms including X, Facebook, Instagram, and YouTube. We´ll count the number of features from the second round of experiments that may appear above the first set of features, providing a set of the features from the newly included apps that now appear above the features from X and Facebook.
Secondly, each prediction was contrasted with the single prediction that Oraculus provides, this is, the predicted result of the experiments will be analyzed with the ’poll of polls’ indicator provided by Oraculus in its "Presidential tracker". This is because we consider the same polls as them and the results of this research can be categorized as a "poll of polls" on its own, but with another set of processing methodologies, now based on social media. This evaluation metric is used since official election results are still unknown and will remain so until June 2.
There is no defined date on which Oraculus bases its monthly prediction. For this, we assigned the features to be summed up before the last day of the testing month (January in this case), e.g. all features are considered and summed up to be used as testing metrics as if Oraculus made its final prediction for January on the 31st.
We do not intend to compete directly with Oraculus.mx. Moreover, we intend to create a completely different methodology to what they do, based now on social media metrics. However, we do consider Oraculus.mx as the guideline to include one poll over another. This is because we believe they provide a necessary filter to know which polls should be considered by the analysis. The extraction of their aggregated polls is used as a way for this paper to save the process of manually looking for polls to include without the proper, unbiased study of each one of them. To the best of our knowledge, this is an innovative approach in the field of election predictions in Latin American countries, as the literature provides no guideline on why a poll may have been considered or not.
To state that a set of features is ’significant’ enough to influence the result of a prediction, we considered the work presented by [14], which took the historic MAE error of 2.7 points to categorize predictions as successful. This metric was measured along the 2 resulting predictions for each model and Oraculus´s result. To conclude significance, we evaluated if a 2.7-point differential existed in the set of results produced by the models which only varied in the networks they were trained upon. If there was such a difference, we declared the inclusion of Instagram and YouTube (apps to be evaluated) significant enough to influence the state of a prediction.
The set of experiments conducted and the retrieval of polls for this work are considered to be around the polls finalized before February 1st.
4 Results
Before exposing the results of the current research, it is important to state that both the retrieval of the data and the analysis of the results started in September 2023, as this was the month in which the two leading candidates were officially named representatives of their respective alliances to compete in the presidential campaign. Jorge Mainez was not included as his data was started to be collected on January 11th, just a day after his official promotion for the national election.
4.1 RQ1. Which social media features are correlated the most with the electoral performance of the selected candidates
Initially, it is crucial to contrast the polls considered throughout the current work. Table 2 presents a summary of the metadata from the included polls in the research. In contrast, Table 3 indicates the number of polls each institution performed until the last round of experiments was performed. High variation is expected from these as electoral preferences change over time.
Candidate | Min | Max | Mean | Median | Std. Dev |
---|---|---|---|---|---|
Sheinbaum | 46 | 66 | 53.77 | 53 | 5.62 |
Gálvez | 13 | 34 | 24.49 | 25 | 4.75 |
Pollster | No. of Polls |
---|---|
Simo | 3 |
Mitofsky | 4 |
Mendoza Blanco Asoc. | 4 |
Enkoll | 4 |
El Financiero | 4 |
Demotecnia | 3 |
Berumen | 2 |
Varela & Asoc. | 1 |
Parametría | 3 |
GEA-ISA | 2 |
Covarrubias y Asoc. | 3 |
Reforma | 1 |
Buendía & Márquez | 1 |
With this, we graph the preference for each candidate as reported by the polls throughout the period we established in previous sections and their reported difference. It is important to add the following figures which cluster together the polls included throughout the analyzed period, as it is easier to observe the number of outliers and the difference that may exist between different values of predictions for polls, see Figure 4:
As pictured in Figure 4 b, the polls seem to agree more on the voting preference for Gálvez, as narrower boxes are depicted in the graph. However, for Sheinbaum, Figure 4 a, presents a wider set of records, especially in January where the polls do not seem to have a concise margin of predictions. It is important to also mention that outliers occur for all the presented pictures, reiterating the fact polls may mark a somewhat clear pattern but not a concise record of results. Similarly, we must note the size of some boxes included and the presence of outliers in the same month.
We can observe a narrower set of records in September concerning the difference between the candidates (Figure 4 c), which indicated a shorter margin between Sheinbaum and Gálvez initially. However, the distance progressively increased in both the margin of predictions and the number of outliers, providing an even less clear image of the real distance between them. As mentioned in previous sections, a wide distance of 25 points between candidates in Estado de México resulted in an eight-point victory for one of them. With this set of results, we can conclude that great margins may not represent the real results of the election, especially with such significant outliers present in the polls, which are completely unaligned from the majority of included ones.
We can observe a growing tendency for the voting preference for Claudia Sheinbaum. The same can be mentioned for the gap reported by the polls between the two candidates. Gálvez on the other hand, appears to have had her highest preference peaking in September of 2023, which can also be referenced in Table 4.
Avg. Preference | Sheinbaum | Gálvez |
---|---|---|
Sep | 51 | 30 |
Oct | 53.57 | 23.71 |
Nov | 52.44 | 23.89 |
Dec | 53.55 | 24.30 |
Jan | 57.75 | 25.38 |
The second phase consisted of examining a given set of metrics across social media for both candidates and aimed to find a correlation between what is observed above depicted by the polls and the online performance of both Sheinbaum and Gálvez. For this, we considered the Pearson correlation between the average polling results (Table 4) and the performance of the candidates in social media with the distinct set of metrics mentioned above.
We must also obtain a general overview of the collected data for each candidate, as seen in Table 5 and Table 6.
Total | Min | Max | Mean | Median | StdDev | |
---|---|---|---|---|---|---|
Xposts | 1679 | |||||
Xcomments | 1066396 | 304 | 46579 | 7254.39456 | 6307 | 4772.62671 |
XRts | 2559811 | 590 | 111756 | 17413.6803 | 16368 | 10430.8728 |
Xlikes | 7275791 | 3239 | 313932 | 49495.1769 | 44487 | 30379.1358 |
FBPosts | 1063 | |||||
Fbreacts | 11592284 | 0 | 261000 | 78859.0748 | 60800 | 56147.6196 |
FBComments | 3128601 | 0 | 88876 | 21283 | 15917 | 16635.8811 |
FBShares | 726546 | 0 | 17781 | 4942.4898 | 4071 | 3004.35102 |
IGPosts | 816 | |||||
IGLikes | 5943466 | 0 | 149731 | 40431.7415 | 37851 | 23312.3129 |
YTPosts | 338 | |||||
YTViews | 32388712 | 0 | 4831000 | 220331.374 | 38400 | 568520.056 |
Total | Min | Max | Mean | Median | StdDev | |
---|---|---|---|---|---|---|
Xposts | 919 | |||||
Xcomments | 681403 | 510 | 13151 | 4635.39456 | 3917 | 2623.89538 |
XRts | 1230276 | 1500 | 25456 | 8369.22449 | 7700 | 3842.93052 |
Xlikes | 3481884 | 4100 | 81468 | 23686.2857 | 22100 | 11596.7216 |
FBPosts | 1248 | |||||
Fbreacts | 7505763 | 2 | 187100 | 51059.6122 | 38400 | 37255.3684 |
FBComments | 931280 | 9500 | 24800 | 6335.2381 | 5474 | 4025.08684 |
FBShares | 707847 | 1279 | 25926 | 4815.28571 | 4422 | 2682.59652 |
IGPosts | 923 | |||||
IGLikes | 4848438 | 0 | 161665 | 32982.5714 | 28016 | 22670.2203 |
YTPosts | 349 | |||||
YTViews | 10688244 | 0 | 847671 | 72709.1429 | 18395 | 131648.559 |
The crucial factor was then to find any correlation that may describe the behavior of the polls based on the social media metrics listed in Tables 5 and 6. To do so, we analyzed the work in [8] in which social media performance (Twitter mentions for different candidates) is correlated to the number of votes these received across their disputes.
For this, we attempted to find a correlation between the metrics mentioned above through their monthly occurrences and the averaged polling results in Table 4. We analyzed which were the features with the most correlation. We sought to visualize them in a correlation matrix, evaluating our results based on the Pearson correlation coefficient r to assess which of those influenced the average polling performances the most.
The results of the correlation experiments are depicted in Figure 5. We can observe the different Pearson correlation coefficients for each feature with the electoral performance of each candidate across time. The features with the highest correlation with the electoral performance mentioned above appear with the darkest tones and at the top of the matrix. We can ignore the Share as this simply corresponds to the that we are aiming to find a correlation with. This coefficient r provides insightful information about how features from commonly ignored social media apps like Instagram can better correlate to the voting performance of a candidate, e.g. the likes per post on Gálvez´s Instagram profile (Figure 5 b) is the single most positively correlated feature that leads and ’tracks’ her polling average. It is the only feature surpassing the r value of 0.7, concluding it would be critically wounding the predicting performance of a model if excluded. As for Claudia Sheinbaum, we observe all engagement metrics for Facebook are features above the r value of 0.7, while the likes of Instagram also equal r to 0.7 (Figure 5 a).
In this way, we evidenced the correlation that features from other social media apps, like Instagram especially, may have on the polling performance of a candidate. Interestingly, none of the features examined from X (Twitter) provided a high Pearson correlation value r to justify its highly adopted usage across research in the field.
4.2 RQ2. Do features from Instagram and YouTube change the outcome of a set of predictions towards the presidential election in Mexico?
For RQ2, we took the lessons from RQ1 to confirm the importance of the features we obtained to be the most correlated with the importance these may have had in modeling experiments.
Both linear regression and the Multi-Layer Perceptron algorithm were tested as described in [4]. The LR algorithm was implemented without any set of parameter tuning. On the other hand, the MLP model included the following parameter setup: one hidden layer with three neurons, L-FBGS as a solver, an alpha set to 0.05, a constant learning rate, and a logistic activation.
As previously discussed, two rounds of experiments for each model were performed: one with only X and Facebook features and another one with features from all platforms mentioned.
The results can be summarized as referenced in Table 7.
Oraculus | LR (XFB) | MLP (XFB) | LR (ALL) | MLP (ALL) | |
---|---|---|---|---|---|
Shainbaum | 64% | 59% | 57% | 64% | 56% |
Gálvez | 30% | 27% | 18% | 41% | 25% |
Before describing them, we can also observe the importance each feature takes in the Linear regression algorithm, as feature importance is clear to visualize. Figure 6 a refers to the feature importance for Sheinbaum coming only from X and FB, while Figure 6 b refers to all features from Sheinbaum. Figure 6 c refers to the feature importance for Gálvez coming only from X and FB, and Figure 6 d refers to all features from Gálvez.
For the Multi-Layer Perceptron model, we will solely use the MAE as the evaluation metric when contrasted with Oraculus´s reported preferences. As evaluated with the Linear Regression results, Table 8 includes the complete set of metrics.
MAE | |
---|---|
LR (X&FB) | 4 |
LR (ALL) | 5.5 |
MLP (X&FB) | 9 |
MLP (ALL) | 6.5 |
As shown, the worst set of results was reported by the MLP model implemented with only those features belonging to X and Facebook solely. More importantly, the inclusion of features from Instagram and YouTube changes the status of a failed prediction (from 9 MAE to 6 MAE) to a successful voting reported preference as described by the MLP (ALL) model. This is an important factor for the development of a predictor, as features from social media applications other than X and Facebook can be a determining factor when evaluating different machine learning models. Concerning the linear regression algorithm, the inclusion of features from Instagram and YouTube only appears to make the predictor 1.5 points worse. This difference is less than the 2.7-point historic MAE result for election margin and is considered, therefore, not significant. Moreover, this does not affect the ’successful’ state of the LR prediction.
Moreover, it is also clear that the Linear Regression model considering only X and Facebook performed better than the iteration considering all social media apps mentioned in this paper. In this case, it is important to point out that while this precise example may argue against the inclusion of other social media features in predicting models, we argue the possible differences in other sets of model experiments (3.5 point difference in MLP for instance) is sufficient to support this inclusion of YouTube and Instagram or their addition into future work in the field over their complete omission. Also, this claim references the different Pearson correlation results we analyzed in previous sections, in which features from Instagram and YouTube had a greater correlation with electoral performance than those of X and Facebook.
When considering the results for Sheinbaum (Figures 6 a and 6 b), we can observe that most of the newly added features from Instagram and YouTube appear to have negative importance to the prediction result. This is, the more negative a feature is the more likely it can be omitted and not impact the result. However, this negative impact is nowhere close to most of the features Facebook already has on the model. In the same sense, the number of Instagram posts appears to be the most influential factor of all the features considered in the second iteration of the model.
Xóchitl Galvez´s results (Figures 12 and 13) are quite in the same direction as Sheinbaum´s: most of the features included even contribute positively to the outcome of the model. While the most important features remain to FB and X, none of the newly considered ones other than the views from YouTube affect the model in a significant way.
5 Discussion
The usage of social media to predict and model political elections is not a novelty. Brito et. al [6] summarized the most important research aiming to do so. However, the usage of social media applications other than X and Facebook has limited the scope of the results and hasn't taken into account the activity on other communication channels like Instagram and YouTube or actual real-world tracking systems like polls [5].
This study has found a correlation between features from highly ignored social media apps and the polling performances of the candidates involved in the 2024 Mexican presidential election. The comparison between these and those belonging to X or Facebook makes it clear some features have not been analyzed enough in the current state of the literature (listed in a previous section), both to model electoral performance off social media and predict the result of an election with online data. Similarly, two modeling approaches evidenced the presence of these features in their feature importance metrics when used to model and predict public preferences.
In this sense, we demonstrated features from Instagram and YouTube better represent the actual behavior of the polls and the polling performance from the different candidates involved to the point that the exclusion of these can make a polling prediction unsuccessful [6], as most of the recent literature has done. The inclusion of unrelated social media indicators (polls for these experiments) and the addition of metrics that do not correspond to either X (Twitter) or Facebook have not been sufficiently explored in the literature.
The goal of the current work is not to discredit the progress made by many existing studies on purely X and Facebook. It aims to bring attention to a possible downside of focusing solely on either of those networks and to credit research that has incorporated them in their experiments. We aim to evidence the need to include data from other social media apps and the possibility of incorporating offline data into future work.
6 Conclusion and Further Research
Throughout the current work, the base was set to use social media to predict the Mexican presidential election. The present research aimed to find a relationship between the presence of the candidates in social media with the results of a considered set of polls and the importance that Instagram and YouTube may have when being considered for modeling election results.
The resulting predictions are ’successful’ as they are close to a considered metric we predefined as the ’poll of polls’ by Oraculus. It is important to clarify that this and the reported predictions are precisely that: predictions of an event that has not happened yet and the outcome of the results may change as the official election result is known on June 2, 2024. However, while the main objective of the current research is to get a similar result to what the election outcome may be, we intend to point out that social media can be used in conjunction with ’real-world’ data (polls) to construct predictors that emulate the behavior of the ’reported’ voting preferences.
Similarly, while we state the current trustworthiness of traditional polling results is declining, we support the idea of their inclusion as real-world indicators by their filtered addition, performed by the Oraculus.mx team.
Finally, we cleared some indicators or features to be more present in the final prediction than others, surprisingly, both Instagram and YouTube are social media networks that influence the used models and are not present in the majority of the current literature around elections, at least when modeled with base-case regression models. This is noticeably important as X, or Twitter has sparked most of the research in the field via a single sentiment classification method, leaving our resulting determinant features out of any possible analysis and therefore, defective or incomplete.
For future research, different models can be included in the predicting phase of the experiment to contrast both the results and the feature importance for each, especially those focusing on time-series forecasting [1]. Also, no filtering process was performed to reduce the number of interactions for any post, and the total number of these was considered, meaning that the usage of both bots to boost candidate interactions and the presence of trolling accounts can influence the traffic a candidate may receive in their social media channels.
Álvarez Mainez´s predictions should also be targeted for future work, as by the time of the present research, not enough data was collected from his social media profiles when considering his recent candidate surge.
References
- Nesreen K Ahmed, Amir F Atiya, Neamat El Gayar, and Hisham El-Shishiny. 2010. An empirical comparison of machine learning models for time series forecasting. Econometric reviews 29, 5-6 (2010), 594–621.
- Amlo Streamer 2023. AMLO supera a Rubius y El Mariana en top 10 de streamers hispanos más vistos. https://www.forbes.com.mx/amlo-desplaza-a-rubius-y-el-mariana-en-top-10-de-streamers-hispanos-mas-vistos/.
- Kellyton Brito and Paulo Jorge Leitão Adeodato. 2022. Measuring the performances of politicians on social media and the correlation with major Latin American election results. Government Information Quarterly 39, 4 (2022), 101745.
- Kellyton Brito and Paulo Jorge Leitão Adeodato. 2023. Machine learning for predicting elections in Latin America based on social media engagement and polls. Government Information Quarterly 40, 1 (2023), 101782.
- Kellyton Brito, Rogério Luiz Cardoso Silva Filho, and Paulo Jorge Leitão Adeodato. 2024. Stop trying to predict elections only with twitter–There are other data sources and technical issues to be improved. Government Information Quarterly 41, 1 (2024), 101899.
- Kellyton Dos Santos Brito, Rogério Luiz Cardoso Silva Filho, and Paulo Jorge Leitao Adeodato. 2021. A systematic review of predicting elections based on social media data: research challenges and future directions. IEEE Transactions on Computational Social Systems 8, 4 (2021), 819–843.
- Cristian Challú, Enrique Seira, and Alberto Simpser. 2020. The quality of vote tallies: Causes and consequences. American Political Science Review 114, 4 (2020), 1071–1085.
- Joseph DiGrazia, Karissa McKelvey, Johan Bollen, and Fabio Rojas. 2013. More tweets, more votes: Social media as a quantitative indicator of political behavior. PloS one 8, 11 (2013), e79449.
- elsoltoluca 2023. Delfina Gómez pide a sus simpatizantes ganar con margen para que no exista duda. https://www.elsoldetoluca.com.mx/local/delfina-gomez-pide-a-sus-simpatizantes-ganar-con-margen-para-que-no-exista-duda-10137707.html.
- Encuestas Electorales 2023. Encuestas Electorales. https://www.ine.mx/encuestas-proceso-electoral-2023/.
- facebooknews 2013. News Feed FYI: A Window Into News Feed. https://www.facebook.com/business/news/News-Feed-FYI-A-Window-Into-News-Feed.
- Fabio Franch. 2013. (Wisdom of the Crowds) 2: 2010 UK election prediction with social media. Journal of Information Technology & Politics 10, 1 (2013), 57–71.
- INEtoluca 2023. Instituto Nacional Electoral. Sistema de Consulta de la Estadística de las Elecciones. Sistema de Consulta de la Estadística de las elecciones del Proceso Electoral 2020-2021. https://siceen21.ine.mx/busqueda/Gubernatura/7/2/2023/2.
- Will Jennings and Christopher Wlezien. 2018. Election polling errors across time and space. Nature Human Behaviour 2, 4 (2018), 276–283.
- Harvey Leibenstein. 1950. Bandwagon, snob, and Veblen effects in the theory of consumers’ demand. The quarterly journal of economics 64, 2 (1950), 183–207.
- Ruowei Liu, Xiaobai Yao, Chenxiao Guo, and Xuebin Wei. 2021. Can we forecast presidential election using twitter data? an integrative modelling approach. Annals of GIS 27, 1 (2021), 43–56.
- Lazaros Oikonomou and Christos Tjortjis. 2018. A Method for Predicting the Winner of the USA Presidential Elections using Data extracted from Twitter. In 2018 South-Eastern European design automation, computer engineering, computer networks and society media conference (SEEDA_CECNSM). IEEE, 1–8.
- Bruce I Oppenheimer, James A Stimson, and Richard W Waterman. 1986. Interpreting US congressional elections: The exposure thesis. Legislative Studies Quarterly (1986), 227–247.
- oraculus 2023. Oraculus. https://oraculus.mx/.
- Alexander Pak, Patrick Paroubek, et al. 2010. Twitter as a corpus for sentiment analysis and opinion mining.. In LREc, Vol. 10. 1320–1326.
- F. Pedregosa, G. Varoquaux, A. Gramfort, V. Michel, B. Thirion, O. Grisel, M. Blondel, P. Prettenhofer, R. Weiss, V. Dubourg, J. Vanderplas, A. Passos, D. Cournapeau, M. Brucher, M. Perrot, and E. Duchesnay. 2011. Scikit-learn: Machine Learning in Python. Journal of Machine Learning Research 12 (2011), 2825–2830.
- PEW Distrust 2024. Public Trust in Government: 1958-2024. https://www.pewresearch.org/politics/2024/06/24/public-trust-in-government-1958-2024/.
- pollsresultsprev 2023. Territorial/ Publimetro: Samuel García sobrepasa a Xóchitl Gálvez y se coloca detrás de Sheinbaum rumbo a la presidencia. https://polls.mx/territorial-publimetro-samuel-garcia-sobrepasa-a-xochitl-galvez-y-se-coloca-detras-de-sheinbaum-rumbo-a-la-presidencia/.
- Bruno Castanho Silva and Sven-Oliver Proksch. 2022. Politicians unleashed? Political communication on Twitter and in parliament in Western Europe. Political science research and methods 10, 4 (2022), 776–792.
- tribunatsanction 2023. La Sala Superior confirmó sancionar a dos encuestadoras por poner en riesgo la equidad en la contienda durante el proceso electoral del Estado de México. https://www.te.gob.mx/front3/bulletins/detail/15292/0.
- Andranik Tumasjan, Timm Sprenger, Philipp Sandner, and Isabell Welpe. 2010. Predicting elections with twitter: What 140 characters reveal about political sentiment. In Proceedings of the international AAAI conference on web and social media, Vol. 4. 178–185.
- Vladimir Vapnik, Esther Levin, and Yann Le Cun. 1994. Measuring the VC-dimension of a learning machine. Neural computation 6, 5 (1994), 851–876.
- A Yavari, H Hassanpour, B Rahimpour Cami, and M Mahdavi. 2022. Election prediction based on sentiment analysis using twitter data. International Journal of engineering 35, 2 (2022), 372–379.
- Robert B Zajonc. 1980. Feeling and thinking: Preferences need no inferences.American psychologist 35, 2 (1980), 151.
- Robert B Zajonc. 2001. Mere exposure: A gateway to the subliminal. Current directions in psychological science 10, 6 (2001), 224–228.
Permission to make digital or hard copies of all or part of this work for personal or classroom use is granted without fee provided that copies are not made or distributed for profit or commercial advantage and that copies bear this notice and the full citation on the first page. Copyrights for components of this work owned by others than the author(s) must be honored. Abstracting with credit is permitted. To copy otherwise, or republish, to post on servers or to redistribute to lists, requires prior specific permission and/or a fee. Request permissions from permissions@acm.org.
ICEGOV 2024, October 01–04, 2024, Pretoria, South Africa
© 2024 Copyright held by the owner/author(s). Publication rights licensed to ACM.
ACM ISBN 979-8-4007-1780-2/24/10.
DOI: https://doi.org/10.1145/3680127.3680213